Machine learning techniques may help identify individualized treatments for critically ill patients
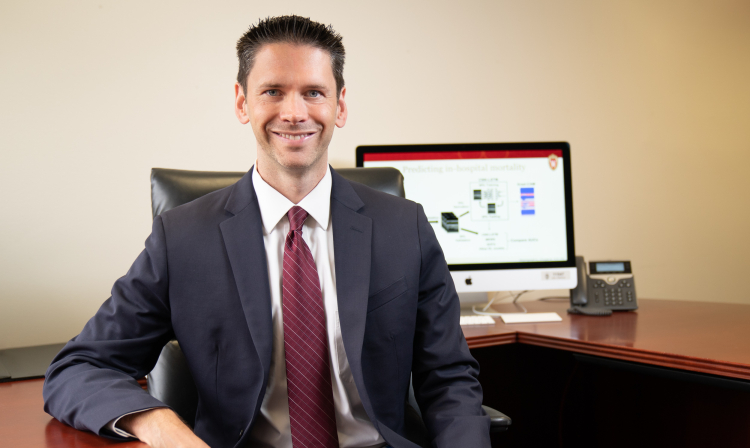
As with many situations in critical care medicine, emergency tracheal intubation—in which a plastic tube is advanced through the mouth, past the vocal cords, and into the trachea—allows no room for trial and error, as the already high risk of cardiac arrest and other complications only increases if intubation is not successful on the first attempt. And while there are several methods of endotracheal tube insertion, there are limited data on which will produce a better result for an individual patient.
Fortunately, data scientists like Matthew Churpek, MD, MPH, PhD, associate professor, Allergy, Pulmonary and Critical Care Medicine, and co-lead of the UW ICU Data Science Lab, are investigating how machine learning—training computers to learn and problem solve through algorithms—might better predict individual treatment outcomes in advance, in turn making those treatments more personalized and effective.
A new study led by Dr. Churpek in collaboration with colleagues at Vanderbilt University and published in the American Journal of Respiratory and Critical Care Medicine demonstrates the potential of machine learning by applying it to data from a clinical trial on intubation techniques.
Bougie or stylet?
The new study examined data from a recent multicenter randomized trial (the BOUGIE trial) of 1,102 patients. The BOUGIE trial compared the effects of the two devices used to guide the endotracheal tube—the stylet (a malleable metal instrument) and the bougie (a flexible plastic rod)—and found that the latter did not increase the likelihood of successful first-time intubation, even among predetermined subgroups of patients.
The BOUGIE trial is typical of clinical trials in that it presents the average treatment effect of two methods across all participants, although the treatment effect for individuals may be different based on their characteristics. Calculating individual treatment effects in a way that goes beyond a single variable—male versus female, etc.—requires a separate analysis that is beyond the scope of most studies. That is where machine learning comes in.
Dr. Churpek and his colleagues wanted to see how certain baseline patient variables—such as difficult airway risk factors, body mass index, and severity of illness—modified the effect of using a stylet or bougie on each patient. To do so, they used the BOUGIE trial data to develop a causal forest model—which resembles thousands of “if/then” causal decision trees.
“For example, a 55-year-old male smoker with high blood pressure has their own individualized treatment effect,” explains Dr. Churpek. “That treatment effect is likely going to be different from the one for a 25-year-old female who’s a nonsmoker with no past medical history. The model learns those individualized treatment effects from the data and uses them to predict these effects in new patients.”
The study’s model identified BOUGIE participants who, contrary to the average treatment effect, would have had better outcomes with the bougie. It also identified those who would have had better outcomes with the stylet. For example, it identified a quarter of patients who would have had a 5% higher rate of first-pass intubation success with a bougie and another quarter of patients who would have had an over 20% higher success rate with a stylet.
Broader implications
These results—and the causal forest model itself—could help identify patients likely to benefit from one method of tracheal intubation over another, which could improve patient outcomes.
“With further validation and testing, one possible use for this model is having it embedded in the electronic health record,” says Dr. Churpek, “where the provider inputs a patient’s baseline characteristics directly into the electronic health record and the model outputs a prediction for them in real time.” This could assist clinicians in making more personalized treatment decisions at the bedside.
More broadly, the study underscores the potential of using machine learning to perform retrospective analysis on clinical trials that had previously been filed away as insignificant.
“Re-investigating these trials could unlock a much more nuanced, richer picture,” Dr. Churpek concludes. “Ultimately, I think, this approach could lead to greater precision medicine and better outcomes for individual patients.”
To learn more about the study and its impact, read the accompanying editorial in the American Journal of Respiratory and Critical Care Medicine, or listen to this podcast episode from the American Thoracic Society featuring Dr. Churpek and the study’s first author, Kevin Seitz, MD, MSc, from Vanderbilt University.
Banner: Dr. Churpek in his office. Credit: Clint Thayer/Department of Medicine.